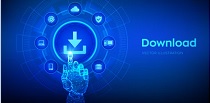
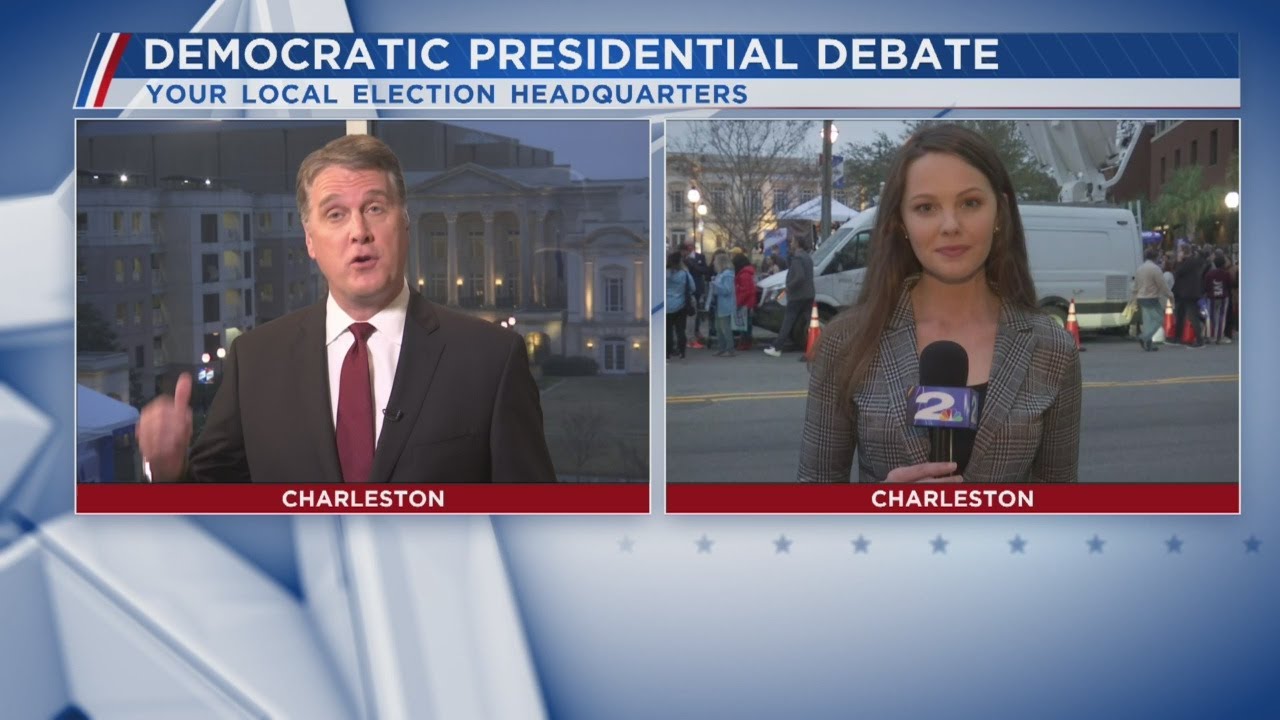
- #HENSLEY ET AL. HYDROLOGICAL PROCESSES 2018 VERIFICATION#
- #HENSLEY ET AL. HYDROLOGICAL PROCESSES 2018 SERIES#
2, a more general assessment of CSD assimilation in Physics of the whole hydrological and hydraulic system, which affects the ( 2017) is unable to properly represent the Relatively paucity of available data, it is shown that the semi-distributed Given the complexity of the basin and the The actual gap between a proof-of-concept study and a real application for theįourth case study presented in Mazzoleni et al., 2017), in order to highlight In-depth assessment of the Bacchiglione River case study (i.e.
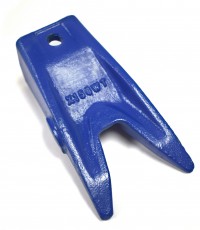
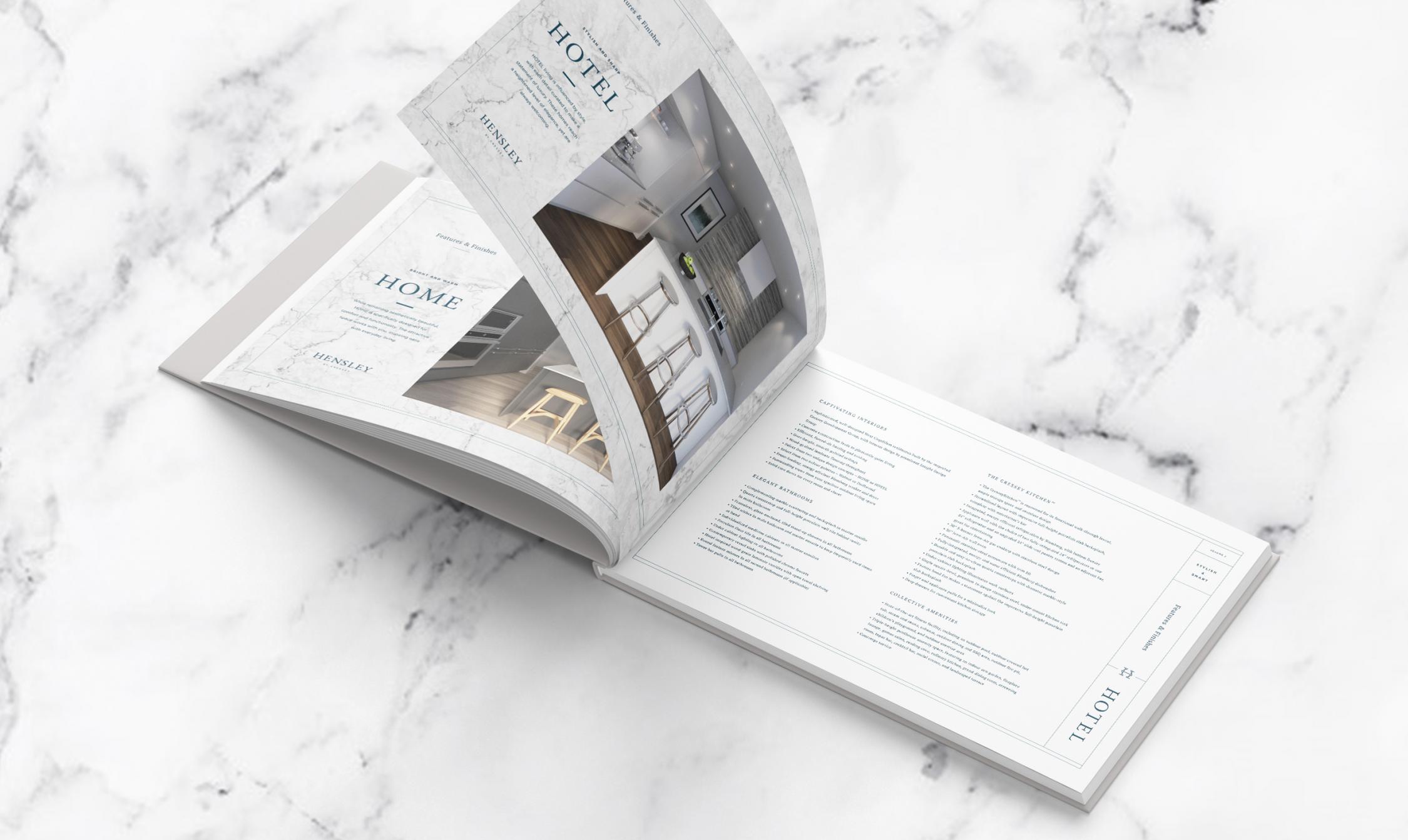
Which are not discussed in their proof-of-concept study. Operational flood forecasting and related to model structural uncertainty, ( 2017) is indeed necessary furthermore, particularĪttention has to be paid to possible drawbacks inherent in the use of CSD for
#HENSLEY ET AL. HYDROLOGICAL PROCESSES 2018 VERIFICATION#
A practical verification of the results by Therefore, the conclusions need to be confirmed using real crowdsourced observations of water level”. not actually measured) CSD,īecause real streamflow CSD were not available at the time of the study.Ĭommenting on this aspect, the authors wrote that “ the developed methodology is not tested with data coming from actual social sensors. In their work, the authors used synthetic (i.e. They also showed that the accuracy of CSD is, in general, more Results showed that assimilation of CSD improves the overall model The issues of uncertainty and irregular arrival frequency of CSD. Reduce uncertainty in forecasting flood hydrographs at downstream sections. Upper part of a basin were assimilated into adaptive hydrological models to In pioneering applications ( Mazzoleni et al., 2015), CSD collected in the
#HENSLEY ET AL. HYDROLOGICAL PROCESSES 2018 SERIES#
In addition, long time series of CSD are unavailable, thus complicatingĮfforts to assess their effectiveness in improving flood prediction. However, the use of CSD posesĬhallenges to modellers since their information content, reliability, arrivalįrequency, and location are a priori unknown ( Le Coz et al., 2016 Walker et al., 2016 de Vos et al., 2017 Smith et al., 2017 Starkey et al., 2017).įor example, the availability of hydrometric data, collected by activeĬitizens in the course of severe flood events, offers a new, exciting chance Providing crowdsourced data (CSD) is gaining increasing attention among Within this general picture, the topic of community-based monitoring aimed at Modellers thus have a responsibility toĭeeply assess the strengths and limitations of model forcing data. It is essential to provide public authorities with the best combination ofĭata and models, and with a robust knowledge of the model behaviour in terms In order to produce the most accurate flood predictions, That are useful for the a priori evaluation of CSD for real-time floodįorecasting and, hopefully, for planning apt design strategies for both modelįlood forecasting has a critical importance as it results in decisions of Of both model structure and forcing data. Requires robust knowledge of the model behaviour and an in-depth assessment Operationalįlood forecasting, which results in decisions of high societal value, States under best-fit conditions can lead to overestimation of theĮffectiveness of CSD assimilation in improving flood prediction. Mimic the actual states of complex systems away from calibration points.Īccordingly, the use of synthetic CSD that are drawn from model internal Structure, internal states of (semi-)distributed models can hardly Result of equifinality, poor model identifiability, and deficiencies in model (semi-)distributed hydrological models deserves further discussion. However, the use of synthetic CSD in conjunction with Irregular frequency of available CSD, and that of data uncertainty, were alsoĭeeply assessed. ( 2017) showed thatĪssimilation of CSD improves the overall model performance the impact of Which is a proof-of-concept study, Mazzoleni et al. The authors used synthetic CSDīecause real CSD were not available at the time of the study. Of crowdsourced data (CSD) into hydrological models to improve the accuracy And crowdsourcing are gaining increasing attention among hydrologists.
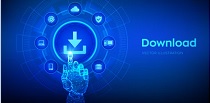