

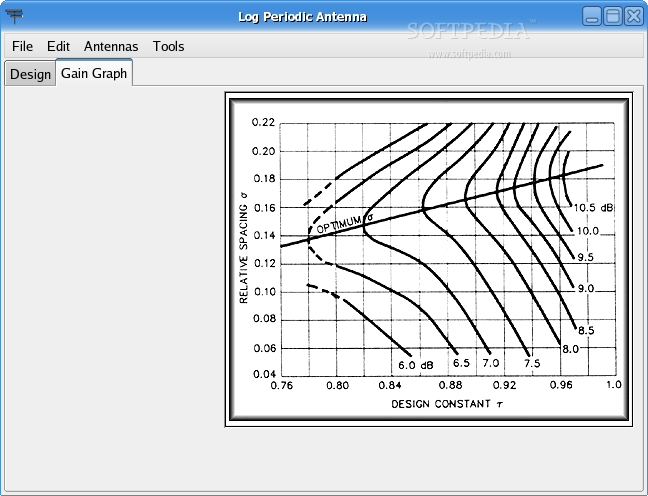
Due to its safety and high time resolution, electroencephalogram (EEG) based BCIs have become popular and various designs using different signals (e.g. The funder had no role in study design, data collection and analysis, decision to publish, or preparation of the manuscriptĬompeting interests: The authors have declared that no competing interests exist.īrain-computer interfaces (BCIs) have potential to help severely disabled people by translating the intentions of subjects into a number of different commands. This is an open access article distributed under the terms of the Creative Commons Attribution License, which permits unrestricted use, distribution, and reproduction in any medium, provided the original author and source are credited.ĭata Availability: All data files are available from the Zenodo database (DOI: 10.5281/zenodo.1146091).įunding: The study has been funded by the Russian Academic Excellence Project ‘5-100’. Received: JAccepted: JanuPublished: January 23, 2018Ĭopyright: © 2018 İşcan, Nikulin. PLoS ONE 13(1):Įditor: Xu Lei, School of Psychology, CHINA The results can be used to improve individual classification performance taking into account effects of perturbations.Ĭitation: İşcan Z, Nikulin VV (2018) Steady state visual evoked potential (SSVEP) based brain-computer interface (BCI) performance under different perturbations. mental, verbal and audio perturbations) on SSVEP-based BCIs. This is the first study that systematically evaluates the effects of natural artifacts (i.e. During the Listening condition relative alpha power in a broad area including central and temporal regions primarily over the left hemisphere correlated negatively with the performance thus most likely indicating active suppression of the distracting presentation of the playback. Therefore we concluded that the drop in the performance is likely to have a cognitive origin. We have not observed significant artifacts in EEG during perturbations in the frequency range of interest except in theta band. The performances were sensitive to the classification method and to the perturbation conditions. During the online condition, Speaking and Thinking decreased moderately the mean classification accuracy compared to Control condition whereas there was no significant difference between Listening and Control conditions across subjects.
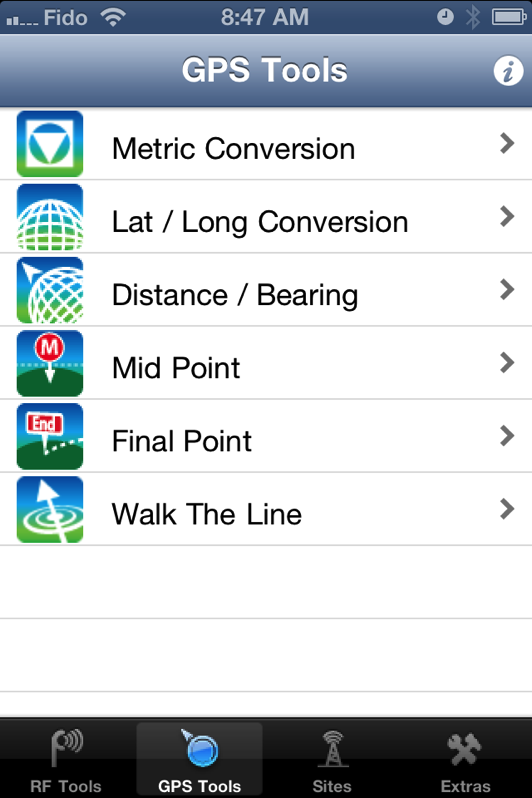
Decision tree, Naïve Bayes and K-Nearest Neighbor classifiers were used to evaluate the classification performance using features generated by canonical correlation analysis. In the online tasks, subjects focused on one of the four circles and gave feedback on the correctness of the classification under four conditions randomized across subjects: Control (no perturbation), Speaking (counting loudly and repeatedly from one to ten), Thinking (mentally counting repeatedly from one to ten), and Listening (listening to verbal counting from one to ten). Specifically we investigated to what extent a drop in performance is related to the degraded quality of EEG signals or rather due to increased cognitive load. In this study, we deliberately introduced different perturbations in order to test the robustness of a steady state visual evoked potential (SSVEP) based BCI. Brain-computer interface (BCI) paradigms are usually tested when environmental and biological artifacts are intentionally avoided.
